New Publication from the SPATRA Project on Rail Buckling Risk Estimation Using Satellite Data and AI
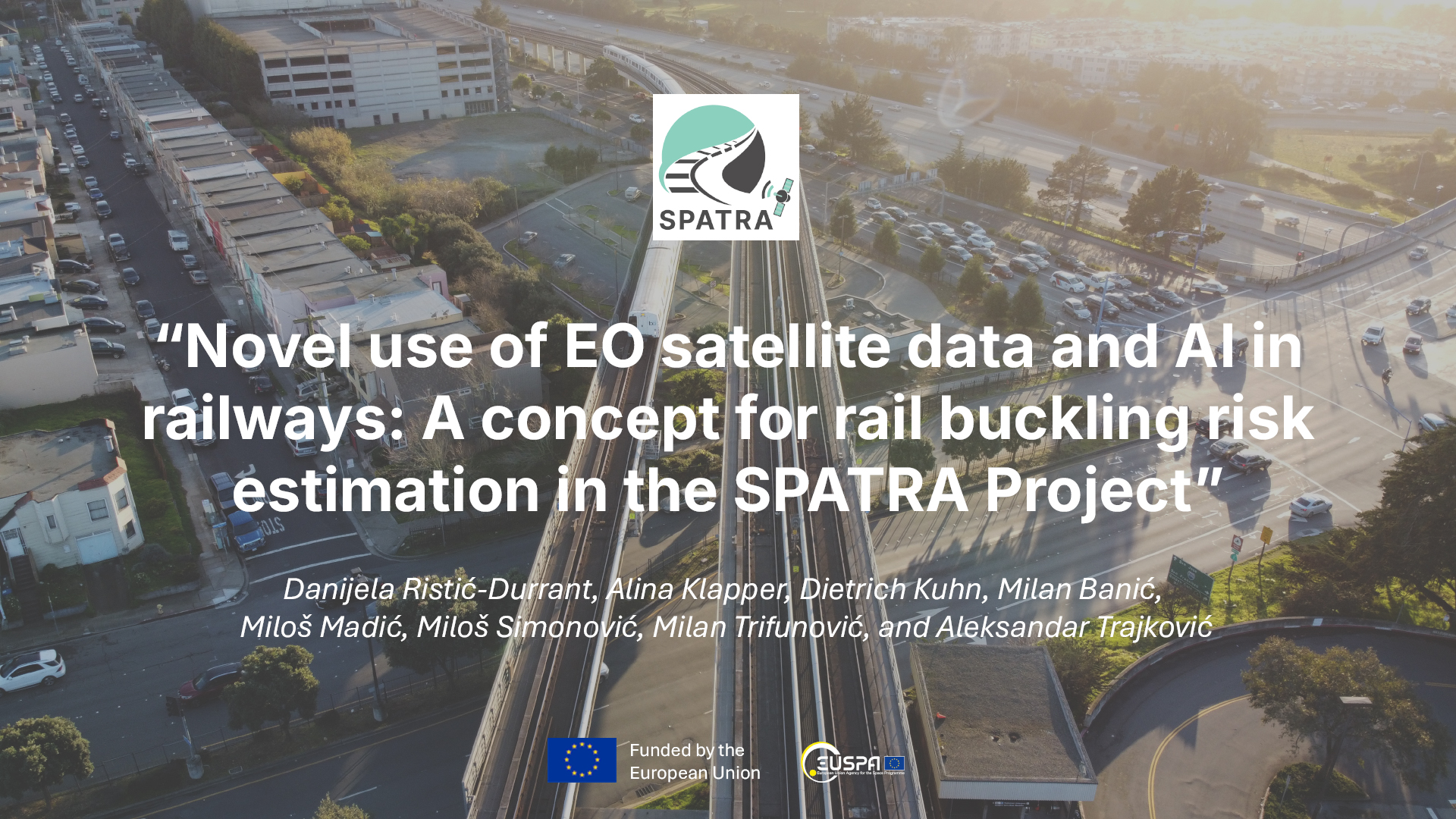
A new paper titled “Novel use of EO satellite data and AI in railways: A concept for rail buckling risk estimation in the SPATRA Project,” authored by Danijela Ristić-Durrant, Alina Klapper, Dietrich Kuhn, Milan Banić, Miloš Madić, Miloš Simonović, Milan Trifunović, and Aleksandar Trajković, has been published in the Proceedings of the 6th International Workshop AI4RAIL 2025, colocated with EDCC 2025.
The paper was accepted for presentation at AI4RAIL2025, and was presented by Danijela Ristić-Durrant during the workshop held in Portugal in April 2025 (AI4RAIL 2025 Program).
The publication introduces a new approach developed within the SPATRA project, focused on leveraging Earth Observation (EO) satellite data and Artificial Intelligence (AI) to improve the estimation of rail buckling risk—a critical challenge for railway infrastructure management in the context of increasing extreme weather conditions.
Innovative Approach to Rail Buckling Risk Estimation
Rail buckling, caused by thermal expansion of tracks during high temperatures, presents a significant safety and operational risk. Traditional monitoring methods are limited in scale and often costly. The SPATRA project proposes an integrated solution using satellite data and AI models to enable large-scale, continuous monitoring and predictive risk assessment.
The concept is built around three main components:
-
High-resolution Land Surface Temperature (LST) data for rail environments
-
Rail Track Temperature Prediction (RTTP)
-
Rail Lateral Displacement Prediction
Each component contributes to a comprehensive system capable of early identification of high-risk rail segments, enabling proactive infrastructure management.
Main Components of the Solution
High-resolution Land Surface Temperature (LST) Data
The first step involves improving the spatial resolution of thermal satellite data. Using machine learning techniques, particularly a step-by-step random forest downscaling method, the resolution of Sentinel-3 land surface temperature data is enhanced from around 1 km to 500 meters or finer.
This allows more detailed temperature monitoring directly over railways, providing essential input for subsequent prediction models.
Rail Track Temperature Prediction (RTTP)
The second component predicts rail track temperatures within a 24-hour forecast window by combining downscaled satellite data, in-situ sensor measurements, weather forecasts, and solar radiation data.
The prediction model is based on XGBoost, chosen for its proven performance in accurately forecasting high rail temperatures.
Rail Lateral Displacement Prediction
The third component uses an artificial neural network (ANN) approach, specifically a multilayer perceptron (MLP), to predict rail lateral displacement based on parameters such as sleeper type, fastener type, track curvature, rail track temperature, track condition, and train operating conditions.
This enables the system to assess the likelihood of track buckling under various conditions, providing actionable insights for rail operators.
For the training and validation of these models, a mobile SPATRA in situ measuring station has been developed. This station collects continuous real-world data on rail temperatures, environmental conditions, and track movements, ensuring that the models are robust and adaptable to different environments.
Advancing Railway Infrastructure Monitoring
The approach developed within SPATRA offers several advantages:
-
Scalable monitoring across large rail networks without reliance on extensive ground-based sensors
-
Early identification of critical risks allowing timely preventive measures
-
Reduction in operational disruptions and maintenance costs
-
Support for infrastructure managers in adapting to climate-related challenges
Future work will focus on real-world validation of the models and further refining their predictive accuracy to support broader adoption across different railway systems.
The study demonstrates how EO data and AI can contribute to developing proactive, cost-effective solutions for improving railway safety and resilience in a changing climate.